Young GI Blog
Clinical Trials 101: Unpacking the Fundamentals - Part 3
June 19, 2023 | Giovanni Marchegiani, Iris Levink, Alberto Balduzzi
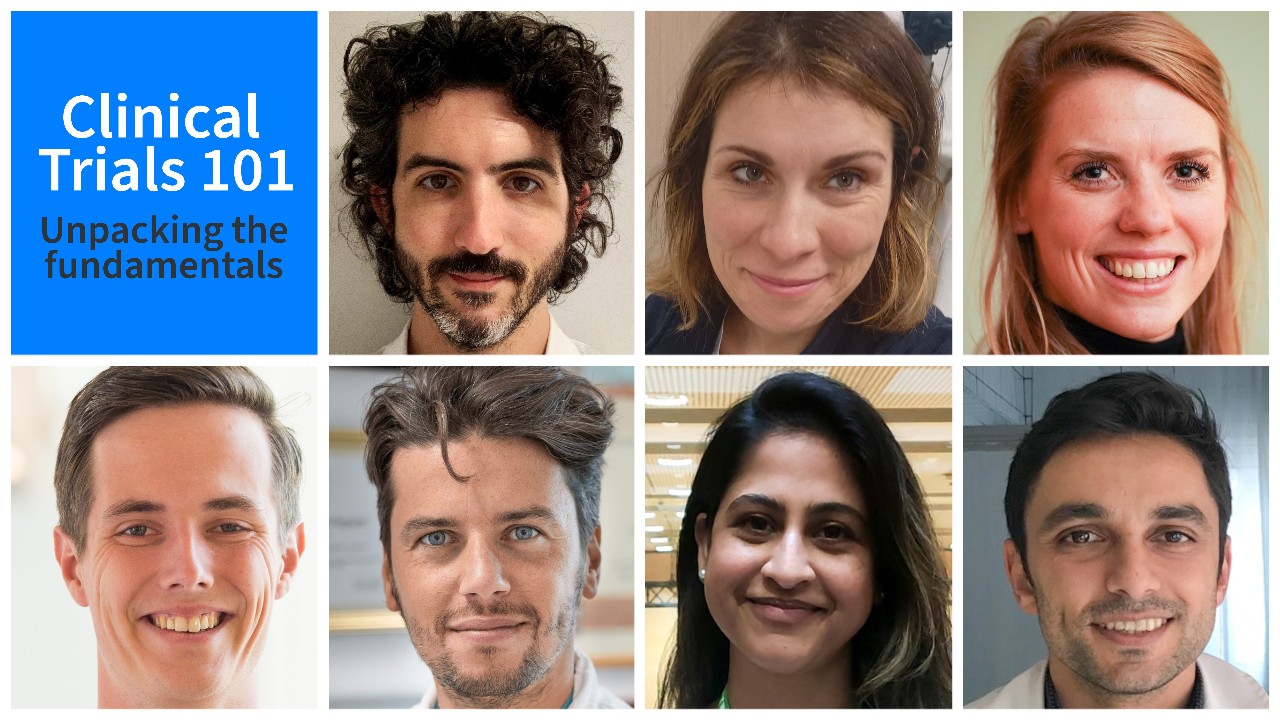
Essential principles in data analysis and interpretation in clinical trials
Clinical trials are an essential and vital part of medical research. They evaluate whether new treatments and interventions are effective, safe, and reliable. The quality of a clinical trial depends on the choices made during the collection, analysis, and interpretation of data. In this blog, we will discuss principles of data analysis and interpretation in clinical trials.
One essential component of clinical trial data analysis is a pre-written statistical analysis plan1. The statistical analysis plan is a detailed document that outlines the statistical methods to be used during the analysis of clinical trial data. It specifies the primary and secondary endpoints, sample size, study populations, and timing of interim and final analyses (including the used statistical tests and variables). Determination of the appropriate sample size calculation is essential. For example, if the sample size is too small the results may not adequately represent the evaluated population. However, a larger sample size might lead to higher costs and efforts for the researchers, as well as potential harm to study participants. The statistical analysis plan should be written before the start of the trial, reviewed by the research team, and approved by a data monitoring committee. It ensures that the analysis is transparent and minimizes the risk of post-hoc analyses that may introduce bias.
The Data Monitoring Committee2 (DMC) is an independent committee that monitors the integrity and safety of a clinical trial. The DMC reviews the accumulating data during the trial and may recommend early termination of the trial in case of safety concerns or if the trial's objectives have not been met. It is strongly encouraged if not mandatory to register the clinical trial on platforms like www.clinicaltrials.gov, as it improves transparency, potentiates new research collaborations, and reduces the risk of publication bias or selective reporting.
Randomization3 is the process of randomly assigning participants to treatment groups. It pursues balanced study groups and secures that differences between groups are due to chance. Blinding involves concealing the treatment allocation from the participants, investigators, and/or outcome assessors ensuring that outcomes can be attributed to the intervention itself and were not influenced by behavior. Randomization and blinding are critical components of a clinical trial design, as they reduce the risk of (detection) bias, enhance the internal validity of the study, and, therefore, increase the likelihood that the observed treatment effects are genuine.
The selection of the type of analyses should be done with caution. An intention-to-treat analysis4 warrants that all randomized patients are included in the final analysis, regardless of the received treatment. It preserves the randomization process and minimizes bias that may arise from missing data or non-compliance. The intention-to-treat analysis is considered the gold standard in clinical trial analysis that evaluates the efficacy of a treatment. In contrast, a per-protocol analysis evaluates the results of all patients that completed the study without major protocol deviations and is particularly useful for the interpretation of non-inferiority trials and the analysis of adverse events of systemic treatment.
Subgroup analysis involves the analysis of a treatment effect within specific subgroups of the study population, like age, sex, or disease severity. Subgroup analysis can provide valuable insights into treatment effects and identify potential effect modifiers. However, it can also lead to false-positive results if it has not been appropriately corrected for multiple testing5. Therefore, subgroup analysis should be considered exploratory or suitable for secondary endpoints and always interpreted with caution.
Efficacy analysis is the process of evaluating the effectiveness of a treatment or intervention. It aims to determine if the investigated treatment has a significant effect on the outcomes of interest in the study population. An efficacy analysis involves comparing the outcomes – for instance, disease progression, symptom relief, or survival – between the treatment group and the control group using appropriate statistical methods. The selection of the statistical test depends on the nature of the distribution of data, the used variables and the study design. For instance, if the authors aim to compare the mean (or median) HbA1c concentration in patients with and without treatment with metformin, the statistical test may be a t-test (or Mann-Whitney U test) or logistic regression. If the authors wish to evaluate if the risk of future development of pancreatic cancer increases with blood HbA1c concentration, a cox regression may be more suitable.
Particularly in trials involving new drugs or treatments, a safety analysis6 may complement the efficacy analysis to identify potential safety issues to inform regulatory decision-making. The safety analysis involves the collection and analysis of adverse events (AEs) and serious adverse events (SAEs) in the treatment and control groups. The use of validated scoring systems, such as Common Terminology Criteria for Adverse Events (CTCAE), supports the interpretation by readers and potentiates the comparison of adverse events between published clinical trials.
The presence of missing data is a common issue in clinical trials and can be caused by various reasons, such as loss-to-follow-up, withdrawal, or death. As missing data may lead to a selection bias and reduce the strength of the study, it should be avoided if possible. There are several techniques to handle missing data, such as multiple imputations, last observation carried forward (LOCF; in case of longitudinal repeated measures), and complete case analysis7. Each method has its advantages and disadvantages. For example, a complete case analysis solely analyses those cases without missing data, which can be beneficial due to its simplicity in analysis and interpretation. However, it risks a significant reduction in statistical power and an imbalance of groups that potentially causes a (selection) bias. Therefore, the choice of method should be based on the nature of the missing data and the research question.
At the time of data interpretation, it is important to note that the statistical significance of the treatment effect does not necessarily imply clinical significance8. Clinical significance refers to the practical or meaningful benefit of the treatment in real-life situations. Therefore, clinical trial results should be interpreted in the context of clinical relevance, considering factors like the risk-benefit ratio, clinical burden, and patient preferences and values. Additionally, external validation of findings is required prior to implementation to evaluate if results can be extrapolated to other cohorts.
Data must be reported in a clear and unbiased manner with the support of tables and figures (if applicable). The Consolidated Standards of Reporting Trials (CONSORT) guidelines9 can also be of assistance. These have been developed as a framework for reporting the results of (randomized) clinical trials in a systematic matter and aim to provide the reader with methodological transparency and, therefore, simplify critical appraisal of the study’s reliability and validity.
In summary, the essential principles in data analysis and interpretation in clinical trials include the adoption of a pre-written statistical analysis plan, a well-considered selection of planned analyses – including sample size calculation and handling of missing data – and the involvement of a data monitoring committee that protects the integrity and safety of the trial. The application of these standards is critical for making informed decisions about the safety and efficacy of new treatments and interventions.
-
References
2. Fleming TR, Demets DL, Roe MT, et al. Data monitoring committees: Promoting best practices to address emerging challenges. Clin Trials. 2017;14:115–123.
3. Boutron I, Estellat C, Guittet L, et al. Methods of blinding in reports of randomized controlled trials assessing pharmacologic treatments: a systematic review. PLoS Med. 2006;3:1931–1939.
4. McCoy CE. Understanding the Intention-to-treat Principle in Randomized Controlled Trials. West J Emerg Med. 2017;18:1075–1078.
5. Alosh M, Huque MF, Koch GG. Statistical Perspectives on Subgroup Analysis: Testing for Heterogeneity and Evaluating Error Rate for the Complementary Subgroup. J Biopharm Stat. 2015;25:1161–1178.
6. Guidelines for Data and Safety Monitoring of Clinical Trials | National Eye Institute Available from: https://www.nei.nih.gov/grants-and-training/policies-and-procedures/guidelines-data-and-safety-monitoring-clinical-trials. Accessed February 18, 2023.
7. Austin PC, White IR, Lee DS, et al. Missing Data in Clinical Research: A Tutorial on Multiple Imputation. Can J Cardiol. 2021;37:1322–1331.
8. Fethney J. Statistical and clinical significance, and how to use confidence intervals to help interpret both. Australian Critical Care. 2010;23:93–97.
9. Bennett JA. The Consolidated Standards of Reporting Trials (CONSORT): Guidelines for reporting randomized trials. Nurs Res. 2005;54:128???132.
Please log in with your myUEG account to post comments.