Chistopher Palmer-Jones is a Senior Fellow in Gastroenterology and IBD at the Royal Free Hospital.
James Lee is a Clinician Scientist Group Leader at the Francis Crick Institute, where he leads the Genetic Mechanisms of Disease laboratory, and an Honorary Consultant Gastroenterologist at the Royal Free Hospital.
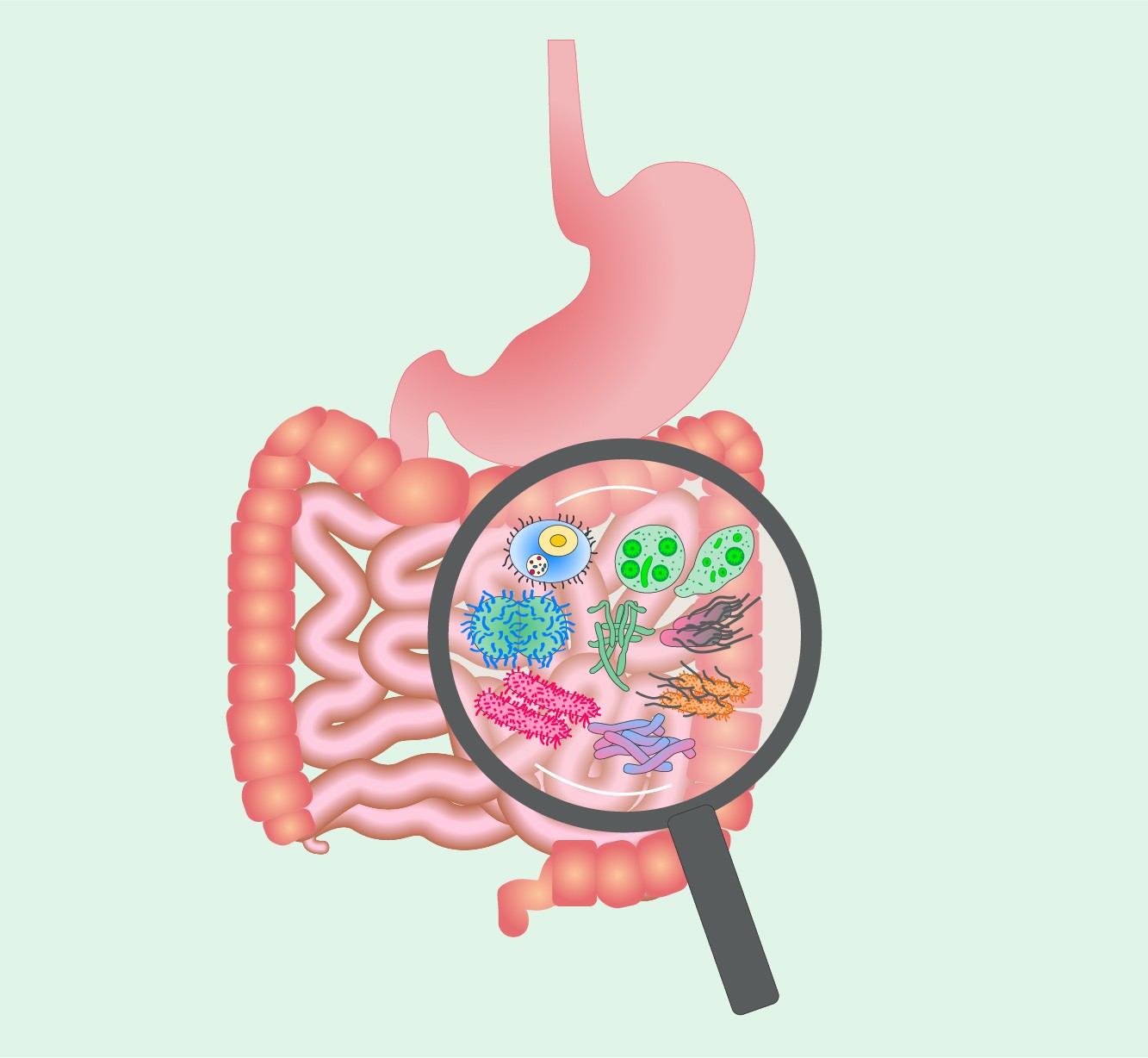
Almost all of the complexity of managing inflammatory bowel disease (IBD) can be traced to the immense heterogeneity of Crohn’s disease and ulcerative colitis.1 Beyond obvious differences in disease distribution and/or behaviour, multiple aspects of IBD are known to differ dramatically – and often unexpectedly – between patients. These include the clinical course of disease and development of complications,2,3 as well as the efficacy, tolerance and toxicity of treatment(s).4 Ensuring that every patient receives the right treatment (for them) at the right time is therefore much easier said than done. Indeed, most current treatment strategies are still based – to some extent – on a “trial-and-error” approach, with recent improvements in clinical care being predominantly driven by tighter monitoring (e.g. “treat to target”) rather than by individualised therapy.5 In other fields of medicine, notably oncology, biomarkers have begun to facilitate a personalised approach to treatment.6,7 Being able to predict – at the time someone is diagnosed with IBD – whether they will require early aggressive therapy and, if so, which treatment would be best for them would represent a major advance.8 For this reason, considerable effort has been invested in developing prognostic (disease course) and predictive (treatment response/side-effects) biomarkers that are both reliable and able to overcome the considerable disease heterogeneity.9
This article highlights common mistakes that are made in the development, interpretation and application of biomarkers in IBD. The discussion is evidence-based, including lessons that have been painfully learned from biomarker studies in other fields. Where evidence is lacking, discussion is based on our experience of developing, evaluating and applying biomarkers in IBD.
@UEG 2023 Palmer-Jones and Lee.
Cite this article as: Palmer-Jones C. and Lee J. C. Mistakes in biomarkers for IBD and how to avoid them. UEG Education 2023; 23: 8-11.
Ilustrations: J. Shadwell.
Correspondence to: [email protected]
Conflict of interest: CPJ has no conflicts of interest. JCL reports financial support for research from GSK, consultancy fees from Abbvie, AgPlus Diagnostics, PredictImmune and C4X Discovery, and is a co-inventor on a patent, “Biomarkers for inflammatory bowel disease”.
Published online: April 6, 2023.
Early attempts to find predictors of disease course in IBD focused largely on clinical parameters. In Crohn’s disease, several features were reported to predict a worse prognosis including early need for steroids, younger age at diagnosis, upper GI involvement, and perianal disease.10-13 Conversely, features including rectal sparing, higher educational level and older age at diagnosis were reported to predict a milder disease course.14 Similar, albeit fewer, studies were conducted in ulcerative colitis (UC), with female gender and smoking reported to reduce the risk of colectomy, while extensive disease and need for steroids/hospitalisation seeming to increase it.15-17
Remarkably, many of these features remain the bedrock of current patient stratification decisions, and yet their predictive performance has long been known to be poor.8 Indeed, even the studies that originally described these associations often documented their poor sensitivity and specificity in predicting prognosis12 – a finding that largely motivated subsequent biomarker efforts. In other words, if clinical features could predict disease course reliably and thereby facilitate personalised therapy, other prognostic biomarkers would never have been needed. Ongoing use of clinical features as stratification tools may well reflect a lack of alternatives. However, it is important to recognise that in isolation, they are not good enough to guide clinical decision-making reliably.
The dictionary definition of the word “prediction” is “a forecast of something that will or might happen in the future”
The dictionary definition of the word “prediction” is “a forecast of something that will or might happen in the future”. The key point, which is worth remembering when critically appraising potential biomarkers, is that prediction relates to something that has not yet happened. Too frequently, predictive and prognostic biomarkers have been described that reflect previous severe disease. For example, some studies reported features including previous bowel surgery, previous need for biological treatment or previous complicated disease as being predictive of more aggressive Crohn’s disease or reduced treatment response rates.18-20 Unfortunately, this is the metaphorical equivalent of predicting that something is flammable because it is on fire! Indeed, by the time such patients have required surgeries and biologics for complicated disease, it should be obvious that they have more aggressive and refractory disease, and no “predictors” are necessary.
Another common mistake in biomarker studies is to assume that a statistically significant association with a particular phenotype automatically equates to being a good predictor ...
Another common mistake in biomarker studies is to assume that a statistically significant association with a particular phenotype automatically equates to being a good predictor. Unfortunately, this is not true – evidenced by the plethora of statistically robust associations with no predictive value.1 To understand why this is – and what we should be looking for instead – it is helpful to consider a non-medical example. If you think about the national team for your favourite sport, they will probably play most of their games in a particular colour shirt/jersey. Consequently, there is likely to be a strong association between supporting that team and owning a replica jersey of that colour (for example, England football supporters will be statistically more likely to own white football shirts than non-England supporters). However, turning this around, would owning a white football shirt be a good predictor of supporting England? The answer is almost certainly, “no”, because while the association undoubtedly exists, it is not sensitive (plenty of England supporters won’t own a white football shirt) nor particularly specific (many other teams play in white, including Germany and Real Madrid just to name two). So how does this apply to IBD biomarkers? In simple terms, we need to look beyond the P value and consider more practical measures of whether a biomarker will be useful, including sensitivity, specificity and – in particular – negative and positive predictive values (NPV / PPV).21 If we do so, then we will quickly realise that even very strong statistical associations may not be predictive. This is why GWAS associations are not individually useful as predictors,22 and why clinical parameters are generally ineffective. Currently, no IBD biomarkers exist that have excellent (and validated) performance for all of these measures (NPV, PPV, specificity and sensitivity). This may not matter, however, since the relative importance of these metrics varies depending on the clinical situation. For example, NPV and sensitivity are recognised to be more important for a prognostic biomarker if the goal is not to miss any patients who might need more aggressive therapy.21 Even with a lower PPV / specificity, a high NPV and sensitivity would mean that a biomarker can effectively identify patients who can safely be managed with a step-up approach due to their low risk of disease progression. Good examples of such biomarkers do exist – for example, the NPV of PredictSURE IBD (PredictImmune Ltd) for predicting the requirement for multiple treatment escalations in Crohn’s disease and UC was 91% and 100% in respective validation cohorts,23 while the NPV of the model developed in the RISK cohort for predicting Crohn’s disease complications was 95%.24
However, it is important to recognise the inherent risks that retrospective studies bring – particularly when using data obtained from recently acquired samples to “predict” previous events ...
It is easy to see the appeal of using retrospective studies to find biomarkers – patient cohorts are generally easier to collect, and so usually larger, and a longer duration of disease can be immediately assessed without needing to wait for many years to elapse. However, it is important to recognise the inherent risks that retrospective studies bring, particularly when using data obtained from recently acquired samples to “predict” previous events. In this situation, a major concern is reverse causation or, in other words, assuming that a result predicts an outcome when it is actually a result of the outcome. For example, there has long been an argument that smoking might cause depression since heavy smokers are more likely to be depressed. But what if people who are depressed smoke to mitigate their low mood? This would reverse the cause-effect relationship, and there is indeed now evidence that heavy smoking is more likely to be a consequence, rather than a cause, of depression.25 In Crohn’s disease, seropositivity to a range of microbial antigens has been repeatedly shown to predict more complicated disease and need for surgery.26-28 While it is clear that a minority of patients do develop antibodies before a diagnosis of IBD,29,30 almost all of the evidence for predicting disease course comes from retrospective studies, in which serological testing was performed on samples collected after many years of disease. As such, what these studies actually show is that patients who have already experienced an aggressive disease phenotype are more likely to be seropositive. A high risk of reverse causation therefore exists, not least because antibodies are only produced following immunological exposure to target antigens.
Consistent with this possibility, several studies have now shown that seropositivity increases with disease duration and that only a minority of patients are seropositive at diagnosis.26,31 One prospective study found a correlation between seropositivity and future complicated disease,32 but the predictive performance was poor and this association was not replicated in subsequent study30 Therefore, it appears that reverse causation may account for much of the apparent predictive effect, with most serologies developing in response to complicated disease, rather than occurring pre-emptively. Further larger studies will be needed in newly diagnosed patients to determine prospectively whether anti-microbial antibodies have any utility as a prognostic biomarker.
Perhaps the most common mistake in current biomarker studies is not performing appropriate validation ...
Perhaps the most common mistake in current biomarker studies is not performing appropriate validation. This is particularly important in studies that combine multiple ‘omic’ technologies because such approaches often assess millions of data points for their individual association with a particular outcome. This is problematic because with enough variables, strong associations are almost inevitable – irrespective of whether they are genuine or spurious.33 Distinguishing true associations from those that have occurred by chance is therefore critical, and largely reliant on independent validation.34 Indeed, a lack of appropriate validation has repeatedly been identified as a reason for biomarker failure in other fields, particularly oncology.35 Why are studies with external validation so rare? In part, this reflects the time and costs associated with recruiting new patient cohorts for biomarker testing, but it probably also reflects the inherent challenges of validation. Many promising biomarkers do not validate in independent cohorts, and even when they do, their performance is typically less impressive than in the initial discovery cohort. This is known as “Winner’s curse”, and reflects the statistical likelihood that newly discovered effects are often overestimated, with subsequent replication attempts producing more modest results. This, however, emphasises why independent validation is so important, and reassuringly an increasing number of studies are now including well-performed validation. For example, a recent description of two disease activity scores – one derived from blood and one from intestinal biopsies – included validation in two cross-sectional cohorts and five pre-existing trial-based cohorts,36 demonstrating how it is sometimes possible to leverage existing datasets to validate biomarkers. It is therefore clear that we should challenge any biomarker whose “validation” relies on its predictive performance in the same cohort in which it was discovered – especially if this discovery was made through a hypothesis-driven comparison (e.g. response vs non-response to a treatment).34 Importantly, lack of validation does not automatically mean that a biomarker is not valid, only that its true predictive value is unknown without additional studies.
Conversely, it is also critical to ensure that validation studies are conducted responsibly and appropriately, using equivalent definitions to those used in discovery studies.34 For example, attempts to validate biomarkers should be sufficiently powered to detect the expected effect – otherwise it is almost impossible to distinguish between a negative result due to insufficient power and a negative result because the biomarker does not work.37 Similarly, the study populations in validation studies should be analogous to those in whom the biomarker was discovered, especially if the biomarker itself could be affected by biological differences between the cohorts.36 For more complex omic biomarkers, it is also crucial to ensure that data analysis is performed carefully and that errors in data handling have not unwittingly contributed to an apparent positive or negative result.38
Aside from challenges relating to their development and validation, these tests will provide a different sort of information to what clinicians are used to seeing ...
Implementing predictive and prognostic biomarkers in clinical practice will not be straight-forward. Aside from challenges relating to their development and validation, these tests will provide a different sort of information to what clinicians are used to seeing. This is because they will not simply provide a readout of current physiology, in the way that standard blood tests do, but rather an indication of how likely something is to happen in the future. Like any forecast, the prediction will not be 100% certain and is likely to involve an estimate of a patient’s relative risk of a particular clinical outcome.1 This means that a good biomarker will still appear to “get it wrong” in some individuals (Figure 1) – simply because the nature of risk means that any predicted outcome, however likely, will not be inevitable. Risk is a concept that is often poorly understood, and even less well explained, although several useful guides have been published39,40 – summarised in Figure 2. For biomarkers to enter clinical practice, we will need to be able to both understand and clearly explain their results – and more importantly what these mean – to our patients and their families.
Figure 1 | Understanding biomarker performance.
A new IBD therapy has a 40% overall response rate. Using a biomarker, it is possible to predict whether patients will respond to this treatment. Predicted responders have an 86% response rate and predicted non-responders have a 15% response rate. The performance characteristics of this biomarker are therefore excellent (detailed in box), but it is important to note that for every 20 patients tested there will still be 2 false negatives and 1 false positive (15% misclassification rate). Created with Biorender.com.
Figure 2 | Top tips for communicating risk.
Thanks to technological advances, there has never been a better time to develop biomarkers for IBD. This is principally because we are no longer limited to small-scale experiments in which only a handful of genes / proteins / metabolites etc. can be measured, but can perform unbiased screens of thousands of potential targets.40 This is a significant advance because often, the best predictors are not the apparent targets but the “unknown unknowns” that would never have been considered for hypothesis-driven studies. However, a drawback of this approach is that with enough variables it is almost impossible to avoid spurious correlations.42 This point is entertainingly, yet clearly, illustrated at https://www.tylervigen.com/spurious-correlations - a website that highlights the sort of entirely spurious correlations that can occur with enough data points (for example, the 99.3% correlation between divorce rates in Maine and US per capita margarine consumption between 2000 and 2009!) Importantly, we can easily identify these examples as spurious because we understand what each variable is, and so can quickly conclude that a causal relationship is implausible. However, spotting a spurious correlation between treatment response and levels of a particular serum metabolite, for example, would be much harder without some knowledge of the underlying biology. For this reason, it is important to consider whether a potential biomarker has biological plausibility, in addition to requiring that it is independently validated (see Mistake 5). This process can also provide useful insights into disease biology. For example, we previously discovered a CD8 T cell transcriptional signature that was present at diagnosis in IBD patients and correlated with subsequent disease course.43 The realisation that this signature reflected differences in T cell exhaustion – the process by which antigen-specific T cells progressively lose their effector functions – not only provided a plausible explanation for the finding, but also offered insights into the biology of prognosis in IBD and a potential treatment target.44 Importantly, this sort of sense check does not always provide clear answers – especially since the biological roles of many genes, for example, are not known – but it should prompt careful scrutiny of any correlations that appear implausible.
Irrespective of specific biomarker successes or failures, it is increasingly clear that we will need a panel of biomarkers to fully realise the potential of personalised medicine in IBD – especially with an expanding therapeutic armamentarium.9 For example, reliably predicting whether a patient will develop a serious side-effect is just as important as knowing whether a patient is likely to respond to the treatment. Similarly, combining prediction of disease course with prediction of treatment response would mean that we can not only identify patients that require more potent therapy, but be able to select the treatment that would be most suitable for them. It is also conceivable that some biomarkers will provide more information when combined with clinical features, or even other biomarkers, than they provide alone. These possibilities can, and should, be formally tested to provide patients with the best chance of receiving the right treatment at the right time.
-
About the authors
-
Biomarkers of IBD briefing
UEG Week
- ‘Neo-epitope protein fragment of calprotectin derived from human neutrophil elastase (CPA9-HNE) is a novel serum calprotectin biomarker of inflammation and disease activity’ session at UEG Week Virtual 2021.
- ‘Circulating MIR-21 and prevalence of IBD in PSC patients’ session at UEG Week 2022.
- Peripheral blood DNA methylation profiles predict response to ustekinumab and show stability during both induction and maintenance treatment in Crohn’s disease’ session at UEG Week Virtual 2021.
- Increased expression of serpin E1, a potential new activity marker, reflects endoscopic activity and therapeutic non-response in inflammatory bowel disease’ session at UEG Week Virtual 2021.
Standards and Guidelines
Please log in with your myUEG account to post comments.